"How Machine Learning is Revolutionizing Industries"
"How Machine Learning is Revolutionizing Industries"
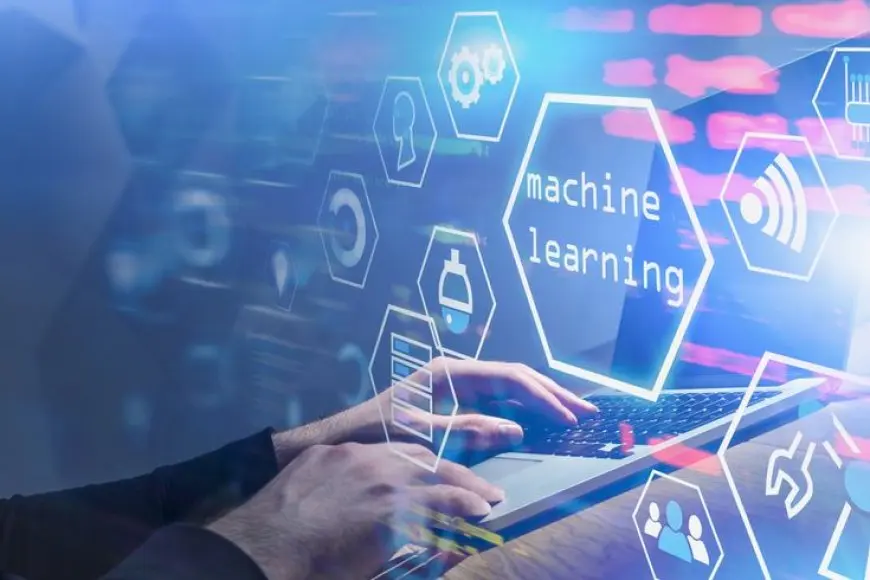
Machine learning (ML) is no longer just a buzzword but a transformative force that is reshaping industries across the globe. A subset of artificial intelligence (AI), machine learning enables systems to learn from data, improve over time, and make decisions without being explicitly programmed. By leveraging vast amounts of data, sophisticated algorithms, and powerful computational models, machine learning is driving innovations in areas such as healthcare, finance, manufacturing, retail, transportation, and many others. The widespread adoption of machine learning has already brought about significant improvements in efficiency, productivity, and decision-making processes, and it is poised to redefine how businesses operate in the coming years.
In healthcare, machine learning is making a profound impact on both patient care and medical research. ML algorithms are increasingly used to analyze medical data, such as patient records, imaging results, and genetic information, to identify patterns and provide more accurate diagnoses. For example, machine learning models can analyze X-ray or MRI images to detect early signs of diseases like cancer, often with greater accuracy than human doctors. Additionally, ML is playing a key role in personalized medicine by helping to design individualized treatment plans based on a patient’s specific genetic makeup. Machine learning also accelerates drug discovery, by analyzing biological data to predict how new drugs will behave, ultimately speeding up the development of life-saving treatments.
The finance industry has also been transformed by machine learning, particularly in areas like fraud detection, algorithmic trading, and credit risk assessment. Banks and financial institutions use machine learning to monitor transactions in real-time and detect fraudulent activity by identifying patterns that deviate from typical behavior. This proactive approach helps prevent financial fraud before it can cause significant damage. In the realm of algorithmic trading, ML models can process vast amounts of market data, identify trends, and make split-second decisions that improve trading strategies and maximize profits. Moreover, machine learning is revolutionizing credit scoring by analyzing non-traditional data, such as social media activity or spending habits, to assess the creditworthiness of individuals and businesses, expanding access to financial services for underserved populations.
In manufacturing, machine learning is driving the industry toward greater automation and efficiency. By integrating ML models into production lines, manufacturers can optimize processes, predict machine failures, and reduce downtime. Predictive maintenance is one of the most significant applications of machine learning in manufacturing; sensors embedded in machinery collect data on performance, which ML algorithms analyze to predict when parts are likely to fail. This allows companies to perform maintenance only when necessary, rather than following a fixed schedule, thus reducing costs and extending the lifespan of equipment. Additionally, machine learning can enhance quality control by identifying defects in products during production, ensuring that only the highest-quality goods reach the market.
Retail is another industry where machine learning is making a dramatic impact. Retailers use machine learning to improve customer experience, optimize inventory management, and personalize marketing efforts. By analyzing customer purchase history, browsing behavior, and preferences, ML algorithms can make personalized product recommendations, leading to higher customer satisfaction and increased sales. Machine learning also plays a key role in demand forecasting, helping retailers predict which products will be in demand at specific times. This allows companies to optimize inventory levels, reduce waste, and ensure that customers can find the products they need when they visit stores or shop online. Furthermore, ML-driven chatbots and virtual assistants are improving customer service by providing quick and accurate responses to customer inquiries 24/7.
In the transportation and logistics sectors, machine learning is revolutionizing how goods and people move around the world. Self-driving cars, trucks, and drones, all powered by machine learning algorithms, are changing the way people and goods are transported. By processing data from sensors, cameras, and GPS, machine learning models enable autonomous vehicles to navigate safely, avoid obstacles, and optimize routes. This has the potential to drastically reduce traffic accidents, increase fuel efficiency, and lower transportation costs. In logistics, ML algorithms are being used to optimize delivery routes, reduce delivery times, and improve fuel consumption. By analyzing real-time data on traffic, weather, and vehicle performance, machine learning models can determine the most efficient delivery schedules, leading to faster, more cost-effective logistics operations.
In the energy sector, machine learning is being used to optimize energy production, consumption, and distribution. ML algorithms can predict energy demand, helping utility companies optimize power generation and distribution to meet the needs of consumers. In renewable energy, such as wind and solar, machine learning helps predict energy generation based on weather patterns, making it easier to integrate renewable sources into the energy grid. Additionally, machine learning models are being used to optimize energy consumption in buildings by learning usage patterns and adjusting heating, ventilation, and air conditioning systems to improve energy efficiency. This not only reduces costs but also contributes to more sustainable energy use.
Despite the many benefits, the implementation of machine learning also presents challenges. One of the main concerns is the need for large amounts of high-quality data to train machine learning models effectively. Without sufficient data, models may not perform well or produce accurate results. Additionally, the complexity of machine learning algorithms can make them difficult to understand and interpret, leading to a lack of transparency in decision-making. This is particularly problematic in industries such as healthcare and finance, where decisions made by machine learning models can have significant consequences for individuals' lives. To address these concerns, researchers are focusing on developing explainable AI techniques that can make machine learning models more transparent and understandable.
Another challenge is the potential for bias in machine learning models. Since these algorithms learn from data, they can inadvertently perpetuate or amplify existing biases present in the training data. For example, a machine learning model used for hiring decisions might favor candidates from certain demographic groups if the training data reflects historical biases in hiring practices. Ensuring that machine learning models are fair and unbiased is a critical area of research and regulation in the field.
In conclusion, machine learning is revolutionizing industries by providing powerful tools for automation, optimization, and decision-making. From healthcare and finance to manufacturing and transportation, machine learning is improving efficiency, enhancing customer experience, and driving innovation. While challenges remain, the future of machine learning holds immense potential for reshaping industries and solving some of the world’s most pressing problems. As technology continues to evolve, machine learning will undoubtedly continue to play a pivotal role in the transformation of industries and the broader economy.